Unleashing the Power of Bayesian Statistical Analysis in Modern Business
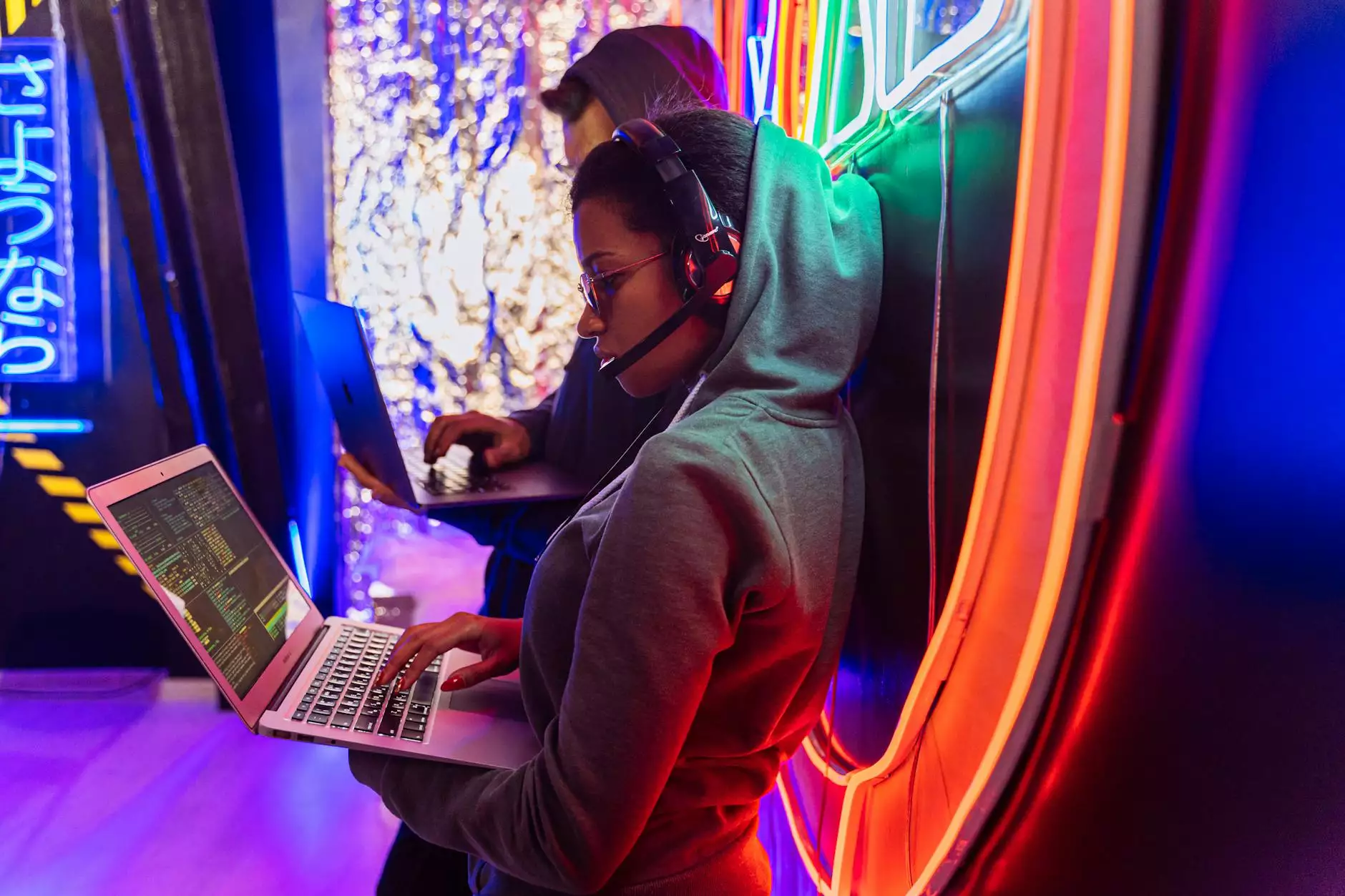
In the burgeoning world of technology and business, organizations seek cutting-edge methodologies to enhance performance, improve decision-making, and bolster security. One such revolutionary approach is Bayesian statistical analysis. This powerful tool is transforming the landscape of IT services and security systems, promising unprecedented insights and a competitive edge in an ever-evolving market. In this article, we will delve deep into the applications of Bayesian statistical analysis within these sectors, showcasing its benefits, methodologies, and numerous real-world applications.
Understanding Bayesian Statistical Analysis
At its core, Bayesian statistical analysis offers a framework for updating beliefs in the face of new evidence. Named after Thomas Bayes, this statistical approach emphasizes the incorporation of prior knowledge into the analysis to yield more accurate predictions and insights. Unlike traditional statistical methods that often rely solely on sample data, Bayesian techniques are inherently flexible, allowing for the adjustment of probabilities as new information becomes available.
Key aspects of Bayesian analysis include:
- Prior Probability: The initial belief before observing the data.
- Likelihood: The probability of observing the data given a particular hypothesis.
- Posterior Probability: The updated belief after considering the new data.
This cyclical process of updating probabilities makes Bayesian analysis particularly useful in fields where uncertainty and risk assessment are prevalent, such as IT services and security systems.
The Role of Bayesian Analysis in IT Services
IT services encompass a wide range of operations, from system administration to software development and technical support. Incorporating Bayesian statistical analysis into IT operations can dramatically enhance efficiency, productivity, and troubleshooting capabilities.
1. Predictive Maintenance
In IT infrastructure management, predictive maintenance is crucial for minimizing downtime and operational disruptions. Bayesian analysis allows businesses to assess the probability of equipment failure based on historical data and current performance metrics. By continuously updating predictions with incoming data, IT departments can implement maintenance protocols proactively, reducing costs and enhancing system reliability.
2. User Behavior Analytics
Another critical application lies in user behavior analytics. With the advent of big data, organizations can analyze vast amounts of user interaction data to uncover patterns. Bayesian methods can be utilized to identify abnormal behavior or deviations from the norm, which may indicate security threats or usability issues. This not only aids in risk mitigation but also enhances user experience through tailored service offerings.
3. Risk Assessment and Management
Effective risk management is synonymous with successful IT operations. By applying Bayesian models, organizations can evaluate various risk scenarios and their potential impacts. This comprehensive analysis allows decision-makers to allocate resources effectively and devise contingency plans that minimize vulnerabilities.
Enhancing Security Systems with Bayesian Analysis
In an age where cybersecurity threats are increasingly sophisticated, leveraging Bayesian statistical analysis in security systems can provide a significant advantage. This approach can enhance threat detection, risk assessment, and incident response.
1. Cyber Threat Detection
Bayesian methods can greatly improve cyber threat detection capabilities by analyzing patterns of behavior across network traffic. By establishing baseline behaviors, security systems powered by Bayesian analysis can detect anomalies that may indicate a potential threat or attack. This proactive stance enables IT security teams to respond swiftly and mitigate potential breaches before they escalate.
2. Fraud Detection
In industries such as finance and e-commerce, fraud detection stands as a paramount concern. Bayesian analysis can aid organizations in identifying fraudulent activities by modeling transaction behaviors and flagging those that deviate significantly from normal patterns. By continuously updating these models with new data, businesses can remain one step ahead of malicious actors.
3. Incident Response Optimization
Following a security breach, a prompt and effective response is essential. Bayesian analysis assists in incident response optimization by assessing the probabilities associated with various response strategies. By evaluating the impact and effectiveness of different actions based on historical incidents, organizations can streamline their response protocols, minimizing damage and downtime.
Implementing Bayesian Statistical Models: Methodologies
To harness the power of Bayesian statistical analysis, organizations must first understand the methodologies involved in its application. Below are several key steps to successfully implement Bayesian models in IT services and security systems.
1. Define the Problem Clearly
Begin by identifying the specific question your analysis aims to answer. A clear problem statement is essential for selecting the appropriate model and data for your Bayesian analysis.
2. Gather Relevant Data
Data is the backbone of any Bayesian analysis. Collect relevant historical data that relates to the problem at hand. This could include transaction records, system logs, user behaviors, and more.
3. Establish Prior Distributions
Design your prior distribution based on prior knowledge or historical data. This sets the foundation for the Bayesian model and helps in the initial prediction.
4. Choose the Likelihood Function
Select a likelihood function that best describes the probability of observing your data given the parameters of interest. This step is crucial for the model's accuracy.
5. Update Your Beliefs
With every new data point, use Bayes' theorem to update your previous beliefs and refine your predictions. This iterative process is what sets Bayesian analysis apart from classical statistics.
6. Validate the Model
Finally, validate your model using a separate dataset to ensure its accuracy and reliability. Continuous refinement may be necessary as new data becomes available.
Moving Forward: The Future of Bayesian Analysis in Business
As technology progresses, the role of Bayesian statistical analysis in business will only grow. With its ability to offer a nuanced understanding of complex systems, organizations that leverage this approach will likely gain a distinct competitive advantage.
Bespoke applications of Bayesian analysis in emerging technologies such as machine learning and artificial intelligence can lead to innovations that were previously unimagined. For instance, self-learning algorithms that adapt based on new data inputs can significantly improve the accuracy of predictions across various domains.
Conclusion
In conclusion, the integration of Bayesian statistical analysis into IT services and security systems presents a momentous opportunity for organizations to enhance their operational efficiency, security posture, and overall decision-making capabilities. By embracing this powerful analytical tool, businesses can navigate the complexities of modern challenges with greater confidence and foresight.
Explore these innovative methods and more at Spambrella.com, where we are committed to providing top-notch IT services & computer repair, as well as robust security systems that leverage the latest in analytical advancements.